Within the Smart digital Ecosystems (SES), the utilization of AI, and Machine Learning (ML) in particular, becomes increasingly prevalent scenarios designed for elevating the user experience of a system’s functional performance. The BIECO approach on building trust in complex ecosystems must also consider the challenge that lies in the assurance of their safety. We use SafeML to assess the reliability and robustness using statistical distance measures.
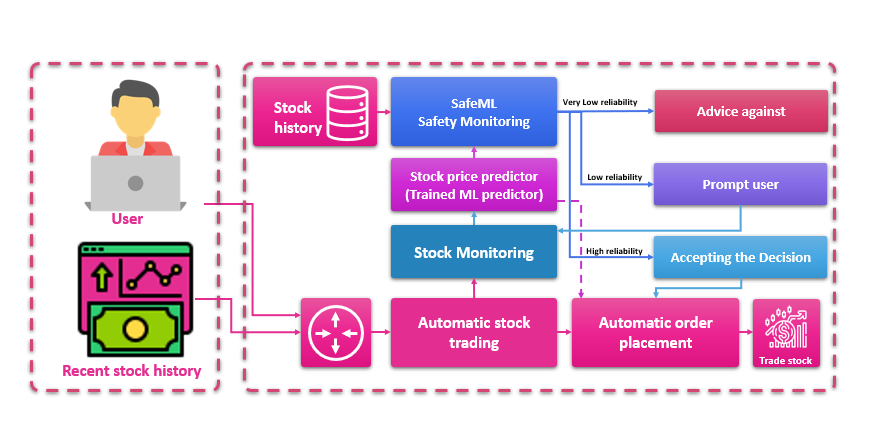
Abstract: Reliability estimation of Machine Learning (ML) models is becoming a crucial subject. This is particularly the case when such models are deployed in safety-critical applications, as the decisions based on model predictions can result in hazardous situations. In this regard, recent research has proposed methods to achieve safe, dependable, and reliable ML systems. One such method consists of detecting and analyzing distributional shifts and then measuring how such systems respond to these shifts. This was proposed in earlier work in SafeML. This work focuses on the use of SafeML for time series data, and on the reliability and robustness estimation of ML-forecasting methods using statistical distance measures. To this end, distance measures based on the Empirical Cumulative Distribution Function (ECDF) proposed in SafeML are explored to measure Statistical-Distance Dissimilarity (SDD) across time series. We then propose SDD-based Reliability Estimate (StaDRe) and SDD-based Robustness (StaDRo) measures. With the help of a clustering technique, the similarity between the statistical properties of data seen during training and the forecasts is identified. The proposed method is capable of providing a link between dataset SDD and Key Performance Indicators (KPIs) of the ML models.
The work is presented in the WAISE workshop of SafeComp 2022. The pre-print version of the work is available here: [2206.11116] StaDRe and StaDRo: Reliability and Robustness Estimation of ML-based Forecasting using Statistical Distance Measures (arxiv.org). The work is also available on Zenodo here: https://zenodo.org/record/6695299